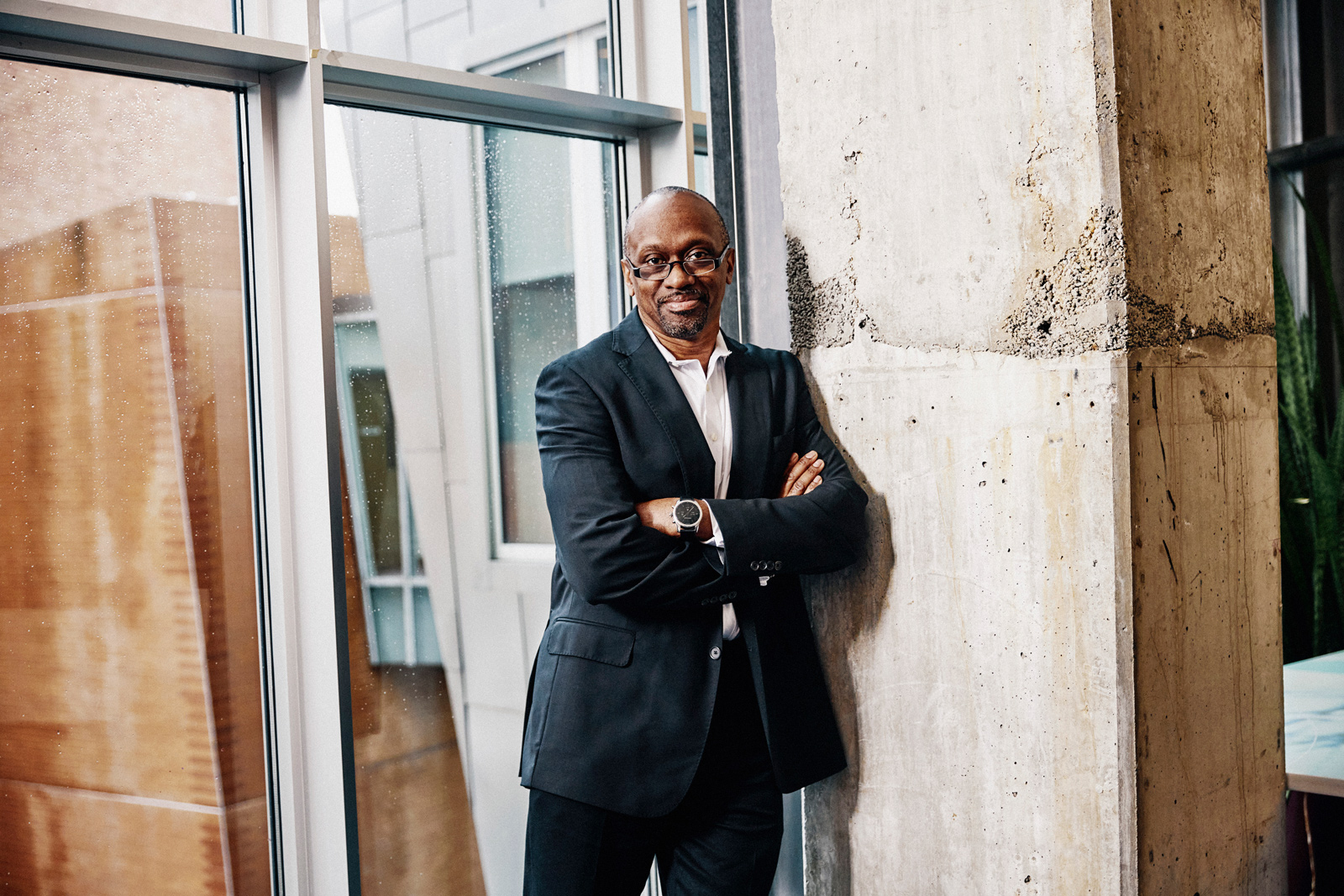
Photo credit: Simon Simard, MIT Spectrum
Professor and physician Collin Stultz, IMES associate director, wants to help heart patients everywhere by applying machine-learning techniques to cardiovascular medicine.
Steve Nadis | MIT Spectrum
Although Collin M. Stultz, MD, loved math throughout high school and his undergraduate years, he headed to Harvard Medical School rather than pursuing pure mathematics, he jokes, to please his parents
Two years later, he began working toward a PhD in biophysics, completing his medical degree and doctorate in the same year. During his internship, residency, and fellowship at Brigham and Women’s Hospital in Boston, Stultz was drawn to cardiology because, he says, “it’s a very evidence-based field.”
Stultz, now the Nina T. and Robert H. Rubin Professor in medical engineering and science and a professor in the Department of Electrical Engineering and Computer Science, joined MIT’s faculty in 2004 and focused his early research on physical chemistry, investigating the proteins involved in various diseases. He showed, for instance, that when collagen—a protein linked to atherosclerosis—degrades and changes shape, it can lead to fatal heart attacks.
Pivoting to machine learning
Stultz, who is also codirector of the Harvard-MIT Program in Health Sciences and Technology and associate director of MIT’s Institute for Medical Engineering and Science, shifted gears after realizing that the patients he was seeing would not derive near-term help from his basic research (IMES is HST's home at MIT). Over a decade ago, he began investigating how patients with cardiovascular disease could benefit from machine-learning techniques, a class of methods in which computer algorithms search for inconspicuous relationships hidden amid large volumes of data. As he continues to explore this strategy, he sees greater potential, although he stresses that “machine learning is still in its nascent phases” in cardiovascular medicine.
One of the greatest opportunities for machine-learning methods lies in what Stultz calls the “sweet spot”—the group of people who fall in between the elderly patients known to have serious heart conditions and the young individuals with no family history of heart disease. In this group, he explains, only a small fraction will die of heart ailments any time soon, yet the total number of deaths will be highest because it constitutes the largest population.
“This is an important group to focus on,” Stultz notes, and available data are sparse. But there are simple ways of gathering data that can tell us, he says, “whom in this cohort we should focus on and whom we don’t have to worry about.” Smartphones, for example, can monitor the number of steps one takes in a day; smartwatches and other wearable devices can measure the electrical activity of the heart in the form of an electrocardiogram (ECG). “Data from these devices contain a lot of information,” Stultz said. “For example, knowing how your heart rate changes with exercise has been shown to be a strong predictor of cardiovascular health.” While the heart rate is just the number of beats per minute, “the ECG can tell you what is going on within each beat, such as whether the heart is getting enough oxygen,” he added. “With the aid of machine-learning algorithms, we can detect subtle changes in the ECG signal that are imperceptible to the human eye.” By making use of strategies like this, he envisions that trips to a physician will become relatively rare—and hospital visits even rarer.
Tracking health threats with algorithms
His research team has recently trained algorithms that can predict pressures inside the left atria of patients with heart failure; as pressure goes up, the threat of congestive heart failure rises. This method is potentially an alternative to the current practice of threading a catheter into the heart, an invasive and sometimes risky procedure. Preliminary results indicate that these AI-abetted predictions were astoundingly good—almost 90% accurate. Clinical trials aimed at validating these results are underway at both the Massachusetts General Hospital (MGH), where Stultz practices cardiology, and at the Boston Medical Center.
Stultz is optimistic that this is just the beginning. “Machine learning will bring more equitable and fairer care for everyone,” he says. “What we want to do, at a high level, is move the hospital to the home setting. If we can help each patient, regardless of their background or where they reside, get the same level of care that a patient at a tertiary care center like MGH receives, then we will be closer to realizing excellent and unbiased care for all patients with cardiovascular disease.”
*Originally published in MIT Spectrum.