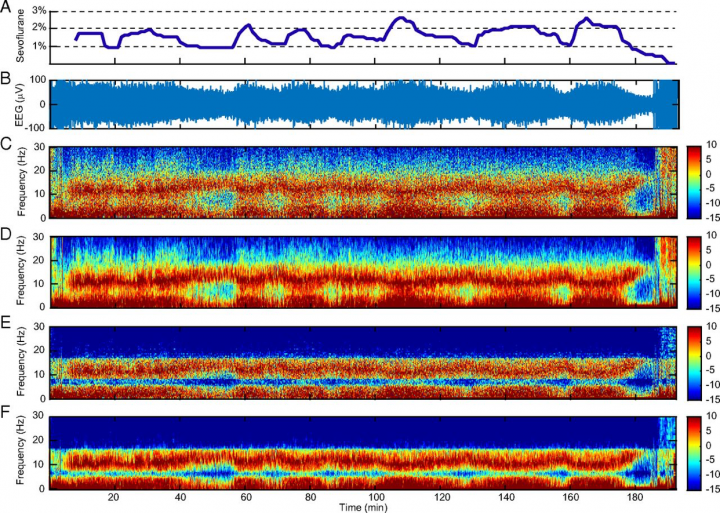
USING A NOVEL ANALYTICAL METHOD THEY HAVE DEVELOPED, MIT RESEARCHERS ANALYZED RAW BRAIN ACTIVITY DATA (B). THE SPECTROGRAM SHOWS DECREASED NOISE AND INCREASED FREQUENCY RESOLUTION, OR CONTRAST (E AND F) COMPARED TO STANDARD SPECTRAL ANALYSIS METHODS (C AND D). IMAGE COURTESY OF SEONG-EUN KIM ET AL.
A NOVEL ALGORITHM ENABLES STATISTICAL ANALYSIS OF TIME SERIES DATA.
Whether it’s tracking brain activity in the operating room, seismic vibrations during an earthquake, or biodiversity in a single ecosystem over a million years, measuring the frequency of an occurrence over a period of time is a fundamental data analysis task that yields critical insight in many scientific fields. But when it comes to analyzing these time series data, researchers are limited to looking at pieces of the data at a time to assemble the big picture, instead of being able to look at the big picture all at once.
In a new study, MIT researchers have developed a novel approach to analyzing time series data sets using a new algorithm, termed state-space multitaper time-frequency analysis (SS-MT). SS-MT provides a framework to analyze time series data in real-time, enabling researchers to work in a more informed way with large sets of data that are nonstationary, i.e. when their characteristics evolve over time. It allows researchers to not only quantify the shifting properties of data but also make formal statistical comparisons between arbitrary segments of the data.
“The algorithm functions similarly to the way a GPS calculates your route when driving. If you stray away from your predicted route, the GPS triggers the recalculation to incorporate the new information,” says Emery Brown, the Edward Hood Taplin Professor of Medical Engineering and Computational Neuroscience, a member of the Picower Institute for Learning and Memory, associate director of the Institute for Medical Engineering and Science, and senior author on the study.
“This allows you to use what you have already computed to get a more accurate estimate of what you’re about to compute in the next time period,” Brown says. “Current approaches to analyses of long, nonstationary time series ignore what you have already calculated in the previous interval leading to an enormous information loss.”
In the study, Brown and his colleagues combined the strengths of two existing statistical analysis paradigms: state-space modeling and multitaper methods. State-space modeling is a flexible paradigm, which has been broadly applied to analyze data whose characteristics evolve over time. Examples include GPS, tracking learning, and performing speech recognition. Multitaper methods are optimal for computing spectra on a finite interval. When combined, the two methods bring together the local optimality properties of the multitaper approach with the ability to combine information across intervals with the state-space framework to produce an analysis paradigm that provides increased frequency resolution, increased noise reduction and formal statistical inference.
To test the SS-MT algorithm, Brown and colleagues first analyzed electroencephalogram (EEG) recordings measuring brain activity from patients receiving general anesthesia for surgery. The SS-MT algorithm provided a highly denoised spectrogram characterizing the changes in power across frequencies over time. In a second example, they used the SS-MT’s inference paradigm to compare different levels of unconsciousness in terms of the differences in the spectral properties of these behavioral states.
“The SS-MT analysis produces cleaner, sharper spectrograms,” says Brown. “The more background noise we can remove from a spectrogram, the easier it is to carry out formal statistical analyses.”
Going forward, Brown and his team will use this method to investigate in detail the nature of the brain’s dynamics under general anesthesia. He further notes that the algorithm could find broad use in other applications of time-series analyses.
“Spectrogram estimation is a standard analytic technique applied commonly in a number of problems such as analyzing solar variations, seismic activity, stock market activity, neuronal dynamics and many other types of time series,” says Brown. “As use of sensor and recording technologies becomes more prevalent, we will need better, more efficient ways to process data in real time. Therefore, we anticipate that the SS-MT algorithm could find many new areas of application.”
Seong-Eun Kim, Michael K. Behr, and Demba E. Ba are lead authors of the paper, which was published online the week of Dec. 18 in Proceedings of the National Academy of Sciences PLUS. This work was partially supported by a National Research Foundation of Korea Grant, Guggenheim Fellowships in Applied Mathematics, the National Institutes of Health including NIH Transformative Research Awards, funds from Massachusetts General Hospital, and funds from the Picower Institute for Learning and Memory.