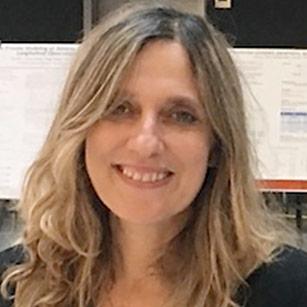
77 Massachusetts Ave.
Cambridge, MA 02139
Degrees
- PhD in Computer Science, Columbia University
Bio
Regina Barzilay is a Delta Electronics professor in the Department of Electrical Engineering and Computer Science and a member of the Computer Science and Artificial Intelligence Laboratory (CSAIL) at MIT. Her research interests are in natural language processing, applications of deep learning to chemistry and oncology. She is a recipient of various awards, including the NSF Career Award, the MIT Technology Review TR-35 Award, Microsoft Faculty Fellowship and several Best Paper Awards at NAACL and ACL. In 2017, she received a MacArthur fellowship, an ACL fellowship and an AAAI fellowship. She received her PhD in Computer Science from Columbia University, and spent a year as a postdoc at Cornell University. She was elected to the National Academy of Medicine in 2023.
More about Professor Barzilay can be found here.
Research Interests
Natural Language Processing
I develop machine learning models that aim to understand and generate natural languages. We are currently witnessing the first generation of NLP tools that have been deployed at scale and are used by millions of people. However, the major component of this success is access to large amounts of training data which machines use to learn mappings between input and output. In many applications and languages, such annotations are not readily available, and are expensive and slow to collect. I am interested in designing algorithms that do not suffer from this annotation dependence. Specifically, we are developing deep learning models that can transfer annotations across domains and languages, that can learn from a few annotated examples by utilizing supplementary data sources, and that can take advantage of human-provided rationales to constrain model structure.
Oncology
Learning to Cure Data collected about millions of cancer patients — their pathology slides, imaging, and other tests — contain answers to many open questions in oncology. Jointly with the MGH collaborators, we are developing algorithms that can learn from this data to improve models of disease progression, prevent over-treatment, and narrow down to the cure. On the NLP side, we are creating databases which record pertinent cancer features extracted from raw documents. On the computer vision side, we are working on deep learning models that compute personalized assessment from mammogram data focusing on early cancer detection.
Chemistry
ML Drug Discovery Today, drug discovery involves practitioners with years of advanced training and is carried out in a trial-and-error, labor-intensive fashion. Our goal is to change a traditional pipeline. In a joint work with chemical engineers and chemists at MIT, we are working on deep learning methods for modeling chemical processes.
Selected Publications
- Bao, Yujia, Shiyu Chang, Mo Yu, and Regina Barzilay, “Deriving Machine Attention from
Human Rationales,” Proceedings of Empirical Methods in Natural Language Processing
(EMNLP), 2018. - Chen, Benson, Regina Barzilay, and Tommi Jaakkola, “Path-Augmented Graph
Transformer Network,” ICML Workshop on Learning and Reasoning with Graph-
Structured Representations, 2019. - Yan, Susu, Enrico Santus, Adam Yala, Leonard Wee, Jason Kim, Santiago A. Lozano-
Calderon, Joseph H. Schwab, Regina Barzilay, Kevin S. Hughes, and Karen De Amorim
Bernstein, “Using Deep Learning Algorithm to Extract Tumour Information from
Pathology and Surgical Notes of Chondrosarcoma Patients,” Proceedings of the
International Conference on the Use of Computers in Radiation Therapy and the
International Conference on Monte Carlo Techniques for Medical Applications (ICCRMCMA),
2019. - Lehman, Eric, Jay DeYoung, Regina Barzilay and Byron C. Wallace, “Inferring Which
Medical Treatments Work from Reports of Clinical Trials,” Proceedings of the North
American Chapter of the Association for Computational Linguistics (NAACL), 2019. - Qian, Yujie, Enrico Santus, Zhijing Jin, Jiang Guo, and Regina Barzilay, “GraphIE: A
Graph-Based Framework for Information Extraction,” Proceedings of the North American
Chapter of the Association for Computational Linguistics (NAACL), 2019. - Schuster, Tal, Ori Ram, Regina Barzilay, and Amir Globerson, “Cross-Lingual Alignment
of Contextual Word Embeddings, with Applications to Zero-Shot Dependency Parsing,”
Proceedings of the North American Chapter of the Association for Computational
Linguistics (NAACL), 2019. - Luo, Jiaming, Yuan Cao, and Regina Barzilay, “Neural Decipherment via Minimum-Cost
Flow: from Ugaritic to Linear B,” Proceedings of the 57th Annual Meeting of the
Association for Computational Linguistics (ACL), 2019. - Jin, Wengong, Kevin Yang, Regina Barzilay, and Tommi Jaakkola, “Learning Multimodal
Graph-to-Graph Translation for Molecule Optimization,” Proceedings of International
Conference on Learning Representations (ICLR), 2019. - Ingraham, John, Vikas K. Garg, Regina Barzilay, and Tommi Jaakkola, “Generative
Models for Graph-Based Protein Design,” ICLR Workshop on Deep Generative Models for
Highly Structured Data, 2019. - Schuster, Tal, Darsh J. Shah, Yun Jie Serene Yeo, Daniel Filizzola, Enrico Santus, and
Regina Barzilay, “Towards Debiasing Fact Verification Models,” Proceedings of Empirical
Methods in Natural Language Processing (EMNLP), 2019. - Fisch, Adam, Jiang Guo, and Regina Barzilay, “Working Hard or Hardly Working:
Challenges of Integrating Typology into Neural Dependency Parsers, “Proceedings of
Empirical Methods in Natural Language Processing (EMNLP), 2019. - Shah, Darsh J., Tal Schuster, and Regina Barzilay, “Automatic Fact Guided Sentence
Modification,” Thirty-Fourth Conference of the Association for the Advancement of
Artificial Intelligence (AAAI), 2020. - Tuan, Luu Anh, Darsh J. Shah, and Regina Barzilay, “Capturing Greater Context for
Question Generation,” Thirty-Fourth Conference of the Association for the Advancement of
Artificial Intelligence (AAAI), 2020.