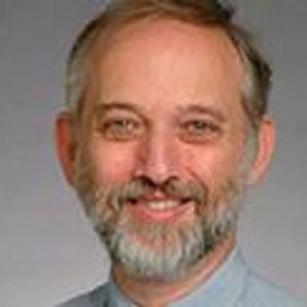
CSAIL
32 Vassar Street
Cambridge, MA 02139
Degrees
- PhD in Information Science, Caltech
- BS in Physics, Caltech
Bio
Peter Szolovits is Professor of Computer Science and Engineering and head of the Clinical Decision-Making Group within CSAIL. His research centers on the application of AI methods to problems of medical decision making and design of information systems for health care institutions and patients. He has worked on problems of diagnosis, therapy planning, execution and monitoring for various medical conditions, computational aspects of genetic counseling, controlled sharing of health information, and privacy and confidentiality issues in medical record systems.
Professor Szolovits’ interests in AI include knowledge representation, qualitative reasoning, and probabilistic inference. His interests in medical computing include Web-based heterogeneous medical record systems, life-long personal health information systems, and design of cryptographic schemes for health identifiers. He teaches classes in artificial intelligence, programming languages, medical computing, medical decision making, knowledge-based systems, computer systems engineering and probabilistic inference.
Prof. Szolovits has served on journal editorial boards and as program chairman and on the program committees of national conferences. He has been a founder of and consultant for several companies that apply AI to problems of commercial interest. He received his bachelor’s degree in physics and his PhD in information science, both from Caltech. Prof. Szolovits was elected to the National Academy of Medicine (formerly the Institute of Medicine) of the National Academies and is a Fellow of the American Association for Artificial Intelligence, the American College of Medical Informatics, and the American Institute for Medical and Biological Engineering and the International Academy of Health Sciences Informatics. He recently served as a member of the National Research Council’s Computer Science and Telecommunications Board and also served as a member of the National Library of Medicine’s Biomedical Library and Informatics Review Committee.
Research
Professor Szolovits’research interests broadly include much of biomedical informatics. He has defined his research interests by the demands of health care and how they could be satisfied by computing approaches. These include creation of decision support tools, machine learning to develop predictive models for alternative interventions, clinical natural language processing to extract information from notes and reports, use of multi-modal data, and means of combining medical knowledge with models learned from data. The Clinical Decision Making Group is dedicated to exploring and furthering the application of technology and artificial intelligence to clinical situations. Because of the vital and crucial nature of medical practice, and the need for accurate and timely information to support clinical decisions, the group is also focused on the gathering, availability, security and use of medical information throughout the human “life cycle” and beyond.
Selected Awards/Societies
- Member of the International Academy of Health Sciences Informatics, 2017
- American College of Medical Informatics: Morris F. Collen Award of Excellence, 2013
- Harvard Medical School Center for Biomedical Informatics: Innovation in Personally Controlled Health Record Infrastructure, 2006
- Member, National Academy of Medicine, 2005
- Fellow, American Institute for Medical and Biological Engineering, 2005
- NASA: Space Act Award, 1995
- Fellow, Association for the Advancement of Artificial Intelligence, 1992
- Fellow, American College of Medical Informatics, 1984
Selected Publications
Most recent publications:
-
163.Ghassemi, M., Wu, M., Hughes, M. C., Szolovits, P., & Doshi-Velez, F.. Predicting intervention onset in the ICU with switching state space models. Proceedings AMIA Summit on Translational Science, 2017, 82–91. PMCID: PMC5543372 (2017)
-
164.Raghu, A., Komorowski, M., Celi, L. A., Szolovits, P., & Ghassemi, M.. Continuous State-Space Models for Optimal Sepsis Treatment – a Deep Reinforcement Learning Approach. http://arxiv.org/abs/1705.08498v1. (2017)
-
165.Luo, Y., Uzuner, Ö., & Szolovits, P. Bridging semantics and syntax with graph algorithms-state-of-the-art of extracting biomedical relations. Briefings in Bioinformatics, 18(1), 160–178. http://doi.org/10.1093/bib/bbw001. PMID: 28472242 (2017)
-
166.Suresh, H., Szolovits, P., & Ghassemi, M. . The Use of Autoencoders for Discovering Patient Phenotypes. arXiv.org. (2017, March 20)
-
167.Dernoncourt, F., Lee, J. Y., & Szolovits, P. Relation Extraction with Convolutional Neural Networks. Semeval. (2017, March 23)
-
168.Dernoncourt, F., Lee, J. Y., & Szolovits, P. NeuroNER: an easy-to-use program for named-entity recognition based on neural networks. arXiv.org. (2017, May 15)
-
169.Lee, J. Y., Dernoncourt, F., & Szolovits, P. . Transfer Learning for Named-Entity Recognition with Neural Networks. arXiv.org. (2017, May 17)
-
170.Luo, Y., Cheng, Y., Uzuner, Ö., Szolovits, P., & Starren, J. . Segment convolutional neural networks (Seg-CNNs) for classifying relations in clinical notes. Journal of the American Medical Informatics Association : JAMIA, 1–6. http://doi.org/10.1093/jamia/ocx090 (2017)
-
171.Suresh, H., Hunt, N., Johnson, A., Celi, L. A., Szolovits, P., & Ghassemi, M. Clinical Intervention Prediction and Understanding with Deep Neural Networks (pp. 1–16). Presented at the mlhc. (2017)
-
172.Luo Y, Szolovits P, Dighe AS, Baron JM. 3D-MICE: integration of cross-sectional and longitudinal imputation for multi-analyte longitudinal clinical data. J Am Med Inform Assoc. 2. doi: 10.1093/jamia/ocx133. PMID: 29202205 (017 Nov 30)
-
173.Weng WH, Wagholikar KB, McCray AT, Szolovits P, Chueh HC. Medical subdomain classification of clinical notes using a machine learning-based natural language processing approach. BMC Med Inform Decis Mak.;17(1):155. doi: 10.1186/s12911-017-0556-8. PMCID: PMC5709846 (2017 Dec 1)
-
174.Yu S, Ma Y, Gronsbell J, Cai T, Ananthakrishnan AN, Gainer VS, Churchill SE, Szolovits P, Murphy SN, Kohane IS, Liao KP, Cai T. Enabling phenotypic big data with PheNorm. J Am Med Inform Assoc. ;25(1):54-60. doi: 10.1093/jamia/ocx111. PMID: 29126253 (2018 Jan 1)
Most recent talks:
-
Computational Identification of Complex Patterns and Temporal Trajectories within Sets of Clinical Laboratory Results. MIT-MGH Grand Challenge Workshop: Diagnostics. 43.12/11/2015.
-
Artificial Intelligence in Medicine: Past, Present, and Future. Pediatrics 2040, Children’s Hospital of Orange County, CA. 4.1/8/2016.
-
Analyzing our Health. (Panel moderator.) MIT IDSS Launch Event. Cambridge, MA. 45.9/23/2016.
-
Interpreting Clinical Narratives: from Linguistic Understanding to Neural Network Modeling. Boston University Natural Language Symposium, Boston, MA. 46.12/13/2017.
-
Toward Automatic Coding and Normalization Strategies. Clinical Natural Language Processing Workshop. Centers for Disease Control and Prevention, Atlanta, GA. 47.12/18/2017.
-
Panel moderator: Benefits of Collaboration. Achieving a Learning Health System for Diagnostic Excellence. University of Michigan, Ann Arbor, MI. 48.3/27/2018.
-
Panel moderator: Challenges, Opportunities, and Progress. AI and Digital Health in Translational Medicine and Clinical Trials. MIT. 49.3/30/2018.
-
Knowledge and Learning: How we moved toward data-driven decision support. Keynote, NorthEast Computational Health Summit. IBM Watson Health, Cambridge, MA. 50.4/27/2018.
A full list of Professor Szolovits publications can be found on his website.
Courses Taught
- HST 956 - SP 2019 - Machine Learning For Healthcare