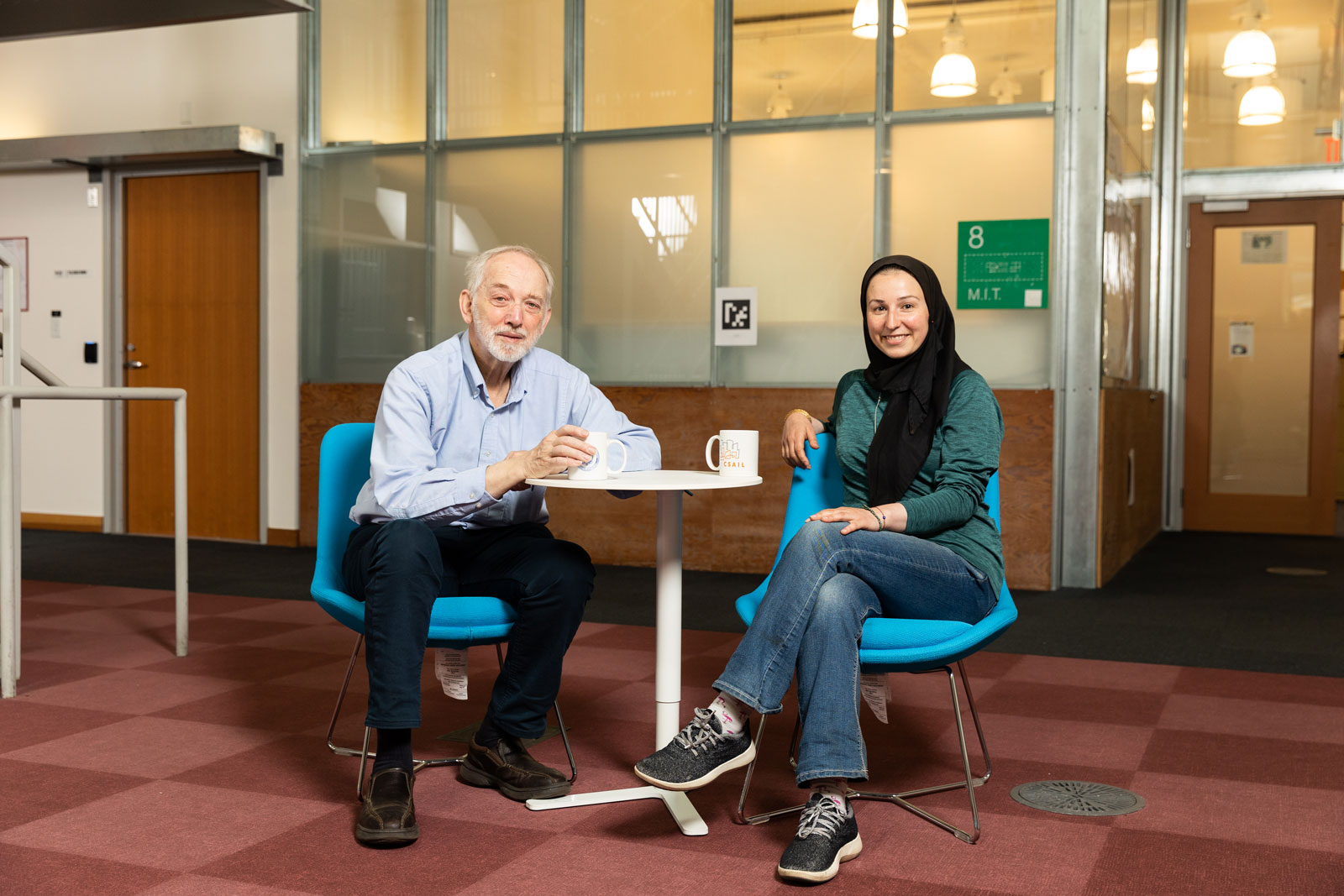
Professor Peter Szolovits and Professor Marzyeh Ghassemi PhD ’17.
PHOTO: JAKE BELCHER
IMES and EECS professor is glad to have crossed academic paths with IMES, computer science and AI faculty Peter Szolovits. The feeling is mutual.
Steve Nadis | MIT Spectrum
During a 2019 interview on Stanford University’s Women in Data Science podcast, Marzyeh Ghassemi PhD ’17, the Hermann Von Helmholtz Career Development Professor, and a core faculty member of the MIT Institute for Medical Engineering and Science (IMES), declared that having a supportive mentor contributed greatly to her success as a graduate student.
“It’s not the project that makes you successful,” she said. “Having the right mentor, and the right people around you, should always be your guiding star.”
In Ghassemi’s case, the right mentor was Peter Szolovits, a core faculty member at IMES, and a professor of computer science and engineering, who served as her PhD and postdoctoral research advisor. Ghassemi first spoke with Szolovits in early 2010 when she was thinking about where to apply for graduate school, then contacted him again in December 2010 after she’d been accepted to MIT and had to decide which lab to join. Szolovits is an expert on applying artificial intelligence (AI) methods to the practice of medicine, which closely aligns with Ghassemi’s interests. During that conversation 13 years ago, Ghassemi’s infant daughter cried repeatedly, and Ghassemi apologized for the interruptions, worried that she was making a bad impression. “That’s OK,” Szolovits assured her. “She’s just asking for her fair share of your time.”
That remark pretty much sealed the deal, Ghassemi recalls. “I realized right then that this was the person for me, someone who would understand that as a nontraditional student—a mother and practicing Muslim who was homeschooled and attended New Mexico State University—I may not be on the same track as everyone else in the lab. He recognized that there are many paths to success.”
Szolovits is grateful that she opted to work in his lab and join the Clinical Decision-Making Group, which he leads in the MIT Computer Science and Artificial Intelligence Laboratory. “She’s a spectacularly good researcher and is immensely energetic,” Szolovits says. “She’s definitely one of the best students I’ve had in my 49 years of teaching at MIT.”
Learning how to become a researcher
Ghassemi’s first big task was to choose a research topic, and Szolovits gave her the time and space she needed for that decision. “Some mentors present students with a list of the top-10 open problems in their field, but that’s definitely not my style,” he says. “I consider learning what to do an important part of the process of learning to become a researcher. I never get in the way of a student who has a good idea by trying to push them in some other direction.”
“She’s a spectacularly good researcher and is immensely energetic,” Szolovits says. “She’s definitely one of the best students I’ve had in my 49 years of teaching at MIT.”
When Ghassemi joined Szolovits’s lab, much of the work involved using natural language processing tools to extract information from clinical notes written by physicians. She wanted to broaden the scope of these inquiries, drawing on additional information—vital sign data, bedside monitor readings, pharmaceutical records, and various lab measurements—to see whether machine-learning algorithms could make more accurate predictions regarding patient outcomes. Acquiring such a multimodal perspective was also one of Szolovits’s goals, and it ended up comprising the heart of Ghassemi’s dissertation project. In a 2014 paper written with Szolovits and others, she demonstrated “that by using vitals, labs, and processed clinical notes, you did better than using any of them alone.” This result gave her confidence that she was on the right path.
Although Szolovits expects to teach his students in the early stages of their work, before long, he expects to learn from them. “And I learned a lot from Marzyeh,” he says, noting that she was the first person to spark his interest in the issue of fairness and bias associated with the incorporation of AI into medicine. “That has become a very important concern,” he points out, both in Ghassemi’s research and in the health care field more broadly.
Previously on the faculty of the University of Toronto, Ghassemi joined MIT in 2020 in the Department of Electrical Engineering and Computer Science—which is housed jointly in the Schwarzman College of Computing and the School of Engineering—and IMES. In addition to her research on effective and equitable uses of machine learning in health care, she now spends much of her time advising students, drawing on the valuable lessons she learned from Szolovits on the art of being a good mentor. “It’s important to encourage students to explore things they personally find interesting rather than just gravitating toward what is ‘hot’ at the moment,” Ghassemi says. “And perhaps the most valuable advice I got from Pete was not to compare myself to other people. ‘You are running a one-person race,’ he told me. ‘Your goal is simply to do the best you can on this path that no one else is on.’”
* Originally published in MIT Spectrum.